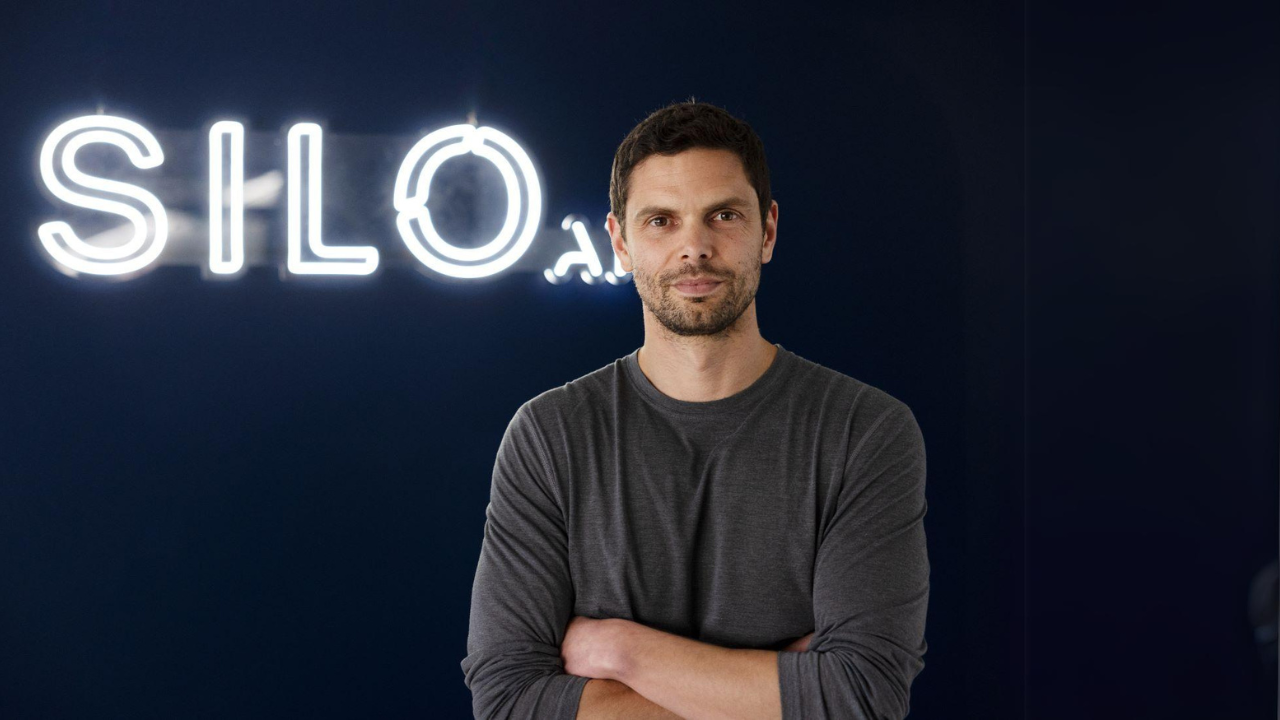
Peter Sarlin from Silo AI on building a European AI flagship, regulating technology and opportunities created by AI
Established in 2017, Silo AI is Europe’s largest private AI lab that partners up with industry leaders to build AI-driven solutions and products to enable smart devices, autonomous vehicles, industry 4.0, and smart cities. We talked to CEO and Co-founder Peter Sarlin about the company’s mission, opportunities created by AI and the challenges of regulating the technology ahead of his talk at sTARTUp Day 2024.
This interview was conducted by Rene Rumberg, a member of the sTARTUp Day communications & marketing team.
This covers everything from working with smart machines and autonomous vehicles with companies like Rolls Royce, Honda, Mitsubishi, forestry and mining machines like Sandvik to segments like smart devices, which includes everything from optimizing image quality on mobile phone cameras to web-scale search engines and medical devices like cancer diagnostics with Philips. The third segment that we work with is what we call smart society which covers a wide range of industries, including finance, retail, and healthcare.
The common denominator is that we're helping companies build and deploy AI at the core of their business and products. This means that we are not an AI consultancy doing small experiments and sprinkling a little bit of AI here and there but we are working on the most challenging, advanced problems, with the purpose of generating long-term value for the client.
So far, we’ve released training and checkpoints of Poro 1 and Poro 2, and will be releasing additional models in the coming months to cover additional languages.
We intend to build European AI infrastructure in line with the European digital sovereignty agenda, and ensure that there exists an open-source LLM that is not only aligned with European values and culture in European languages, but also functions as an open-source base that allows European companies to create value on top of, allowing the value to be created in Europe.
These models are trained using extensive, carefully selected data for tasks like prediction or classification. Our current understanding is centered around generative pre-trained transformer (GPT) models, like ChatGPT. At their core, these are word prediction tools. Large language models process vast amounts of text, often trillions of word tokens from the web, to learn how to predict the next word in a sentence. They also develop broader capabilities that enhance their word prediction, oftentimes called emergent properties.
It’s important to note that this technology doesn't equate to consciousness as we understand it, or necessarily even intelligence. Therefore, it's not a direct step towards artificial general intelligence or superintelligence. Instead, it represents a specific application of AI that, despite its limitations, continues to offer significant value, much like the machine learning technologies we've used for many years.
What we have seen during the past 10 years is a significant increase in value creation with AI around sensors and sensor data, covering camera sensors, LiDAR, laser and radar data, etc. That is, for example, the underlying data empowering autonomous vehicles, but also a very large number of other applications that rely on sensors. The maturity of that technology has been evolving since the full self-driving hype. Even though the original goal of full self driving has not been realized, our cars are filled with features that come out of self-driving technology so the technological development has already created value in that context, and the same is true in many other industries.
I see the same happening now around language technology. If you look at the ChatGPT wave, it has happened with the intent to build Artificial General Intelligence or AGI as OpenAI has widely communicated. I don't think we or they know at this point what is actually going to take us to AGI, but even if we don't reach AGI through LLMs, the work on the technology around language is creating value and is being integrated into products, like GitHub Copilot, Bing, Teams and of course wide range of others.
Just like a self-driving vehicle is a vehicle that has AI in it, GitHub Copilot is GitHub that has AI as part of it, increasing the ultimate value generated by the product.
What I see happening now is wide-scale deployment of AI technology, especially large language models, into software products, where language is an interface. This will have an impact on many industries, from healthcare, finance, insurance and software development to a wide range of other industries.
What sectors are the most promising for investors looking to invest in AI-based companies?
You could look at it from the perspective of sectors and verticals or from a horizontal perspective.
As an investor, there's plenty of opportunity in tooling infrastructure, the horizontal space where you're building enabling technology from various types of MLOps infrastructure – how you actually deploy, operate, run, and monitor machine learning in production – to how you build foundation models and allow others to benefit from them.
As to verticals, I believe there are opportunities in every single industry out there. The core question is, do you succeed in investing in a product that is creating enough value to penetrate the market? This is typically not an AI question but a product question. I would be careful about investing in pureplay AI companies, but look for companies that are deep enough in the vertical in the specific industry and have an opportunity to elevate their product with the help of AI.
However, I do think that there's a significant risk related to the fact that we now have technology that passes the Turing test, which implies that we as end users can’t distinguish whether we are interacting with a human or a machine. And I think that is going to lead to a very large amount of information that we do not know whether it's accurate or defined in a certain way with a certain purpose. This on its own implies a certain level of risk that needs to be regulated.
The challenges come from the latest legislative addition during this last year, which was the treatment of the foundation models. It remains to be seen how exactly they will be regulated, but I think it can be more problematic for regulators to define and eventually for companies to comply with these rules. This is because you then start to move into definitions of what you regulate that sort of resemble systems and methods, which are very difficult to define.
The EU wants to regulate AI more compared to some other regions, especially Asia. Won’t this lead to the risk that we’ll be lagging behind in innovation?
I think the EU AI Act has been taking good steps toward the use-case specific angle to regulation. We already have industry-specific regulation in place before the EU AI Act so it's nothing new. Various industries have been regulated in various ways and, of course, AI is a component to that.
I think Europe, but overall various jurisdictions, need clarity. The positive impact from getting the regulation in place is that it allows companies in Europe to have more certainty when they make investment decisions in this technology, as they know what is the regulatory environment within which they operate.
Peter Sarlin will talk about building a European AI flagship in a fireside chat with Rebecka Löthman Rydå on Day 2 of sTARTUp Day on January 26.
Check out the festival schedule.
To start with, could you tell us what exactly is Silo AI doing and what sets you apart from modern companies?
Silo is a private AI lab with a fairly unique team – more than 300 AI experts, over half of whom have a PhD in AI-related fields. We work with globally leading brands in a wide range of industries, helping them to build and deploy AI at the core of their products. We are offering a combination of AI models, AI tooling, and AI services to help companies succeed.This covers everything from working with smart machines and autonomous vehicles with companies like Rolls Royce, Honda, Mitsubishi, forestry and mining machines like Sandvik to segments like smart devices, which includes everything from optimizing image quality on mobile phone cameras to web-scale search engines and medical devices like cancer diagnostics with Philips. The third segment that we work with is what we call smart society which covers a wide range of industries, including finance, retail, and healthcare.
The common denominator is that we're helping companies build and deploy AI at the core of their business and products. This means that we are not an AI consultancy doing small experiments and sprinkling a little bit of AI here and there but we are working on the most challenging, advanced problems, with the purpose of generating long-term value for the client.
In December, together with the University of Turku, you launched Poro – the first checkpoints of a family of multilingual open-source large language models (LLMs), covering all official European languages and code. Can you tell us more about that?
We are working on one of the largest open-source multilingual language models called Poro after the Finnish word for reindeer. We're building it on LUMI, Europe's most powerful supercomputer and the third most powerful supercomputer in the world, using a record amount of data and compute for open-source language models.Our goal is to build an open-source, multilingual, large language model that covers all official European languages and reflects European values and culture.
So far, we’ve released training and checkpoints of Poro 1 and Poro 2, and will be releasing additional models in the coming months to cover additional languages.
We intend to build European AI infrastructure in line with the European digital sovereignty agenda, and ensure that there exists an open-source LLM that is not only aligned with European values and culture in European languages, but also functions as an open-source base that allows European companies to create value on top of, allowing the value to be created in Europe.
The term ‘large language model’ or LLM went into the mainstream when ChatGPT was released a year ago, however, I’ve seen many people ask about what it really means. How would you explain in layman's terms what LLMs are?
Large language models are a form of advanced machine learning systems or neural networks, which is not an inherently new technology, but one that has been in development for half a century. While their size and architecture have evolved, the underlying principles remain largely the same.These models are trained using extensive, carefully selected data for tasks like prediction or classification. Our current understanding is centered around generative pre-trained transformer (GPT) models, like ChatGPT. At their core, these are word prediction tools. Large language models process vast amounts of text, often trillions of word tokens from the web, to learn how to predict the next word in a sentence. They also develop broader capabilities that enhance their word prediction, oftentimes called emergent properties.
It’s important to note that this technology doesn't equate to consciousness as we understand it, or necessarily even intelligence. Therefore, it's not a direct step towards artificial general intelligence or superintelligence. Instead, it represents a specific application of AI that, despite its limitations, continues to offer significant value, much like the machine learning technologies we've used for many years.
What would you say are those sectors that would benefit the most from the implementation of AI?
We tend to have an umbrella term as AI to define value creation but it's usually more complicated. Different AI technologies contribute to different sectors.What we have seen during the past 10 years is a significant increase in value creation with AI around sensors and sensor data, covering camera sensors, LiDAR, laser and radar data, etc. That is, for example, the underlying data empowering autonomous vehicles, but also a very large number of other applications that rely on sensors. The maturity of that technology has been evolving since the full self-driving hype. Even though the original goal of full self driving has not been realized, our cars are filled with features that come out of self-driving technology so the technological development has already created value in that context, and the same is true in many other industries.
I see the same happening now around language technology. If you look at the ChatGPT wave, it has happened with the intent to build Artificial General Intelligence or AGI as OpenAI has widely communicated. I don't think we or they know at this point what is actually going to take us to AGI, but even if we don't reach AGI through LLMs, the work on the technology around language is creating value and is being integrated into products, like GitHub Copilot, Bing, Teams and of course wide range of others.
I think it's important to have a product lens on when you look at industries. It's not only about industries and use cases in those industries, but about who has scalable software solutions that are creating value in those industries that are then elevated with AI.
Just like a self-driving vehicle is a vehicle that has AI in it, GitHub Copilot is GitHub that has AI as part of it, increasing the ultimate value generated by the product.
What I see happening now is wide-scale deployment of AI technology, especially large language models, into software products, where language is an interface. This will have an impact on many industries, from healthcare, finance, insurance and software development to a wide range of other industries.
What sectors are the most promising for investors looking to invest in AI-based companies?
You could look at it from the perspective of sectors and verticals or from a horizontal perspective.
As an investor, there's plenty of opportunity in tooling infrastructure, the horizontal space where you're building enabling technology from various types of MLOps infrastructure – how you actually deploy, operate, run, and monitor machine learning in production – to how you build foundation models and allow others to benefit from them.
As to verticals, I believe there are opportunities in every single industry out there. The core question is, do you succeed in investing in a product that is creating enough value to penetrate the market? This is typically not an AI question but a product question. I would be careful about investing in pureplay AI companies, but look for companies that are deep enough in the vertical in the specific industry and have an opportunity to elevate their product with the help of AI.
There’s been a lot of talk about the existential threat of and hence the need to regulate AI. What is your take on that?
I am not one of the proponents of discussions around existential risks, I don't think that is a relevant discussion given the current state of technology. Of course, we don't know what the technology will be in the future but the technology we have today is not exposing us to existential risks.However, I do think that there's a significant risk related to the fact that we now have technology that passes the Turing test, which implies that we as end users can’t distinguish whether we are interacting with a human or a machine. And I think that is going to lead to a very large amount of information that we do not know whether it's accurate or defined in a certain way with a certain purpose. This on its own implies a certain level of risk that needs to be regulated.
In your opinion, what will be the best way to approach regulating AI?
I do believe that as with other technologies, AI should also be regulated. I've always been a proponent of industry-specific, application-specific and use-case-specific regulation, rather than generic horizontal AI regulation. It's very difficult to define systems and methods, or even agree on what AI is. As AI on its own isn't really a technology, it's more of a conceptual definition of a set of different technologies with a common sort of purpose.The challenges come from the latest legislative addition during this last year, which was the treatment of the foundation models. It remains to be seen how exactly they will be regulated, but I think it can be more problematic for regulators to define and eventually for companies to comply with these rules. This is because you then start to move into definitions of what you regulate that sort of resemble systems and methods, which are very difficult to define.
The EU wants to regulate AI more compared to some other regions, especially Asia. Won’t this lead to the risk that we’ll be lagging behind in innovation?
I think the EU AI Act has been taking good steps toward the use-case specific angle to regulation. We already have industry-specific regulation in place before the EU AI Act so it's nothing new. Various industries have been regulated in various ways and, of course, AI is a component to that.
I think Europe, but overall various jurisdictions, need clarity. The positive impact from getting the regulation in place is that it allows companies in Europe to have more certainty when they make investment decisions in this technology, as they know what is the regulatory environment within which they operate.
Peter Sarlin will talk about building a European AI flagship in a fireside chat with Rebecka Löthman Rydå on Day 2 of sTARTUp Day on January 26.
Check out the festival schedule.
Articles you might also like:
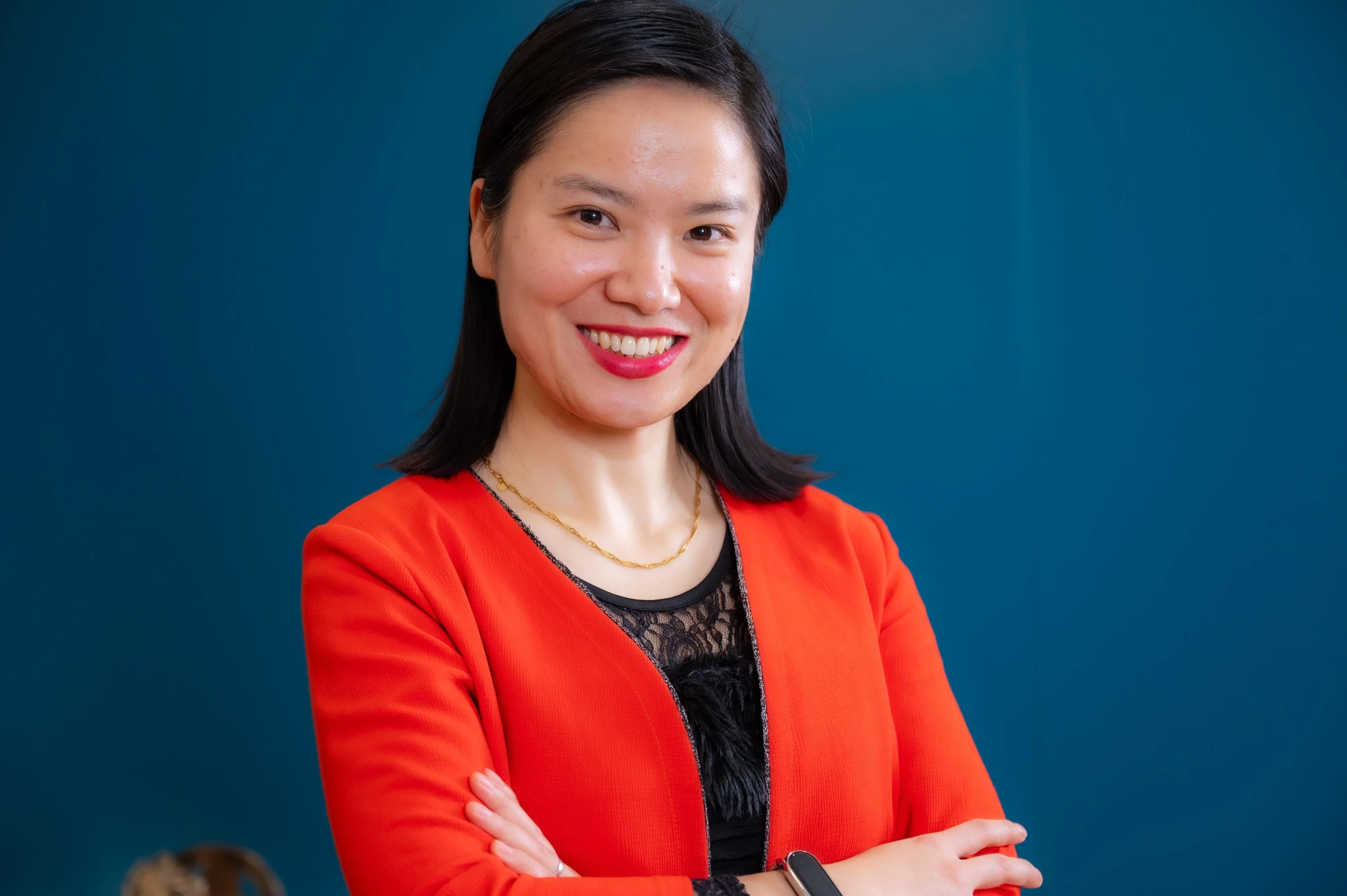
Shaping the Future with Technology: A Conversation with Dr. Christina Yan Zhang
28.01.2025
As technology rapidly reshapes industries and societies, few people have been at the forefront of this transformation, quite like Dr. Christina Yan...
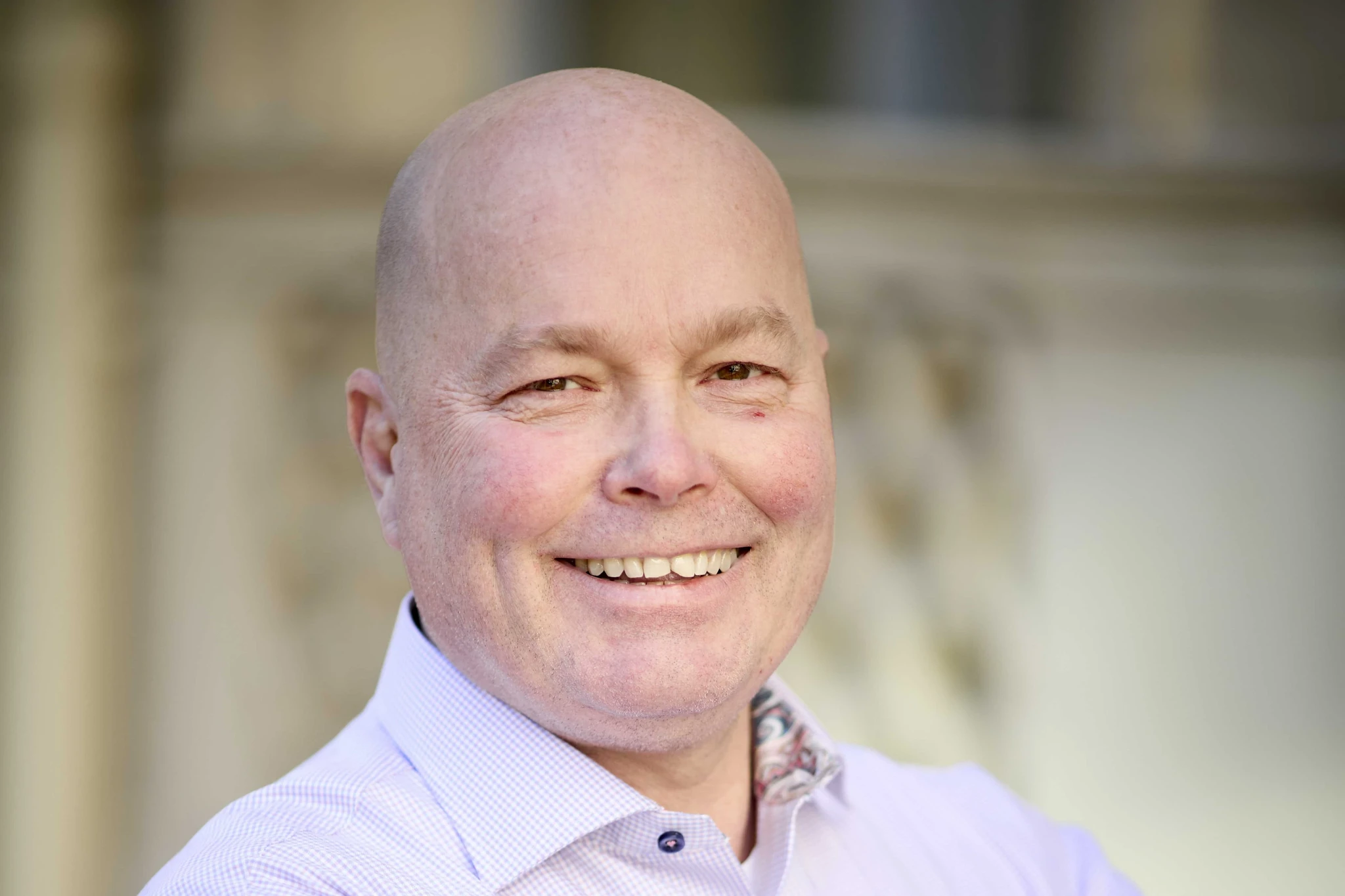
From Business Angel to Rocket Science: Ted Elvhage on Investing, Innovation, and the Future of Deep Tech
24.01.2025
Ted Elvhage, a pioneering investor with a portfolio spanning over 45 companies, shares his journey from enterprise software entrepreneur to co-founding Stockholm...